Seminar Data-Science II (Empirical Studies)
IS 723 for Master students (M.Sc. MMM, M.Sc. WiPäd)
Lecturer | Prof. Dr. Markus Strohmaier, Marlene Lutz, Maximilian Kräutner |
Course Format | Seminar |
Offering | HWS |
Credit Points | 6 ECTS |
Language | English |
Grading | Written report (50%), oral presentation (40%) and discussion (10%) |
Examination date | See schedule below |
Information for Students | The course is limited to 12 participants. The registration process is explained below. |
Contact
For administrative questions, please contact office.strohmaier. uni-mannheim.de
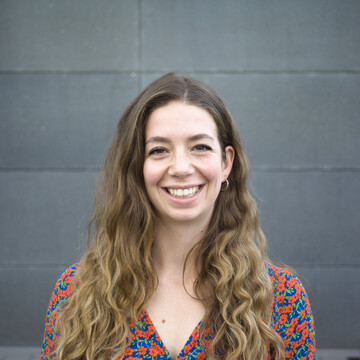
Marlene Lutz
L 15, 1–6
3rd floor – Room 323
68161 Mannheim
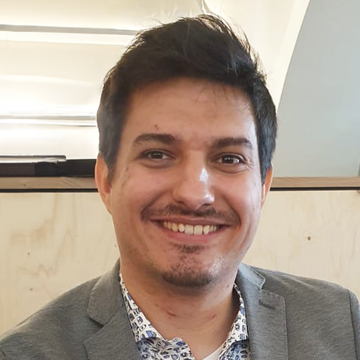
Maximilian Kreutner
L 15, 1–6
3rd floor – Room 322
68161 Mannheim
Course Information
Course Description
The achievement of the learning goals is pursued by practicing on the basis of personally assigned in-depth scientific topics as well as by actively participating in the presentation dates. The organizer will choose subject areas within the field of Data-Science (see Topics) and provide scientific papers to students to work through.
Previous participation in the courses offered by our chair are recommended.
Topics
This seminar will be split into two main topic blocks. Every student will be assigned a research paper from only one of these blocks to work on. Yet, it is expected that students also actively participate in discussion on papers from the other topic blocks after they have been presented.
When applying for this seminar, please indicate whether you would be interested in only one or both topic blocks. The two topics we are going to discuss in the HWS 2024 are:
- Representativeness of Language Models. Large Language Models (LLMs) are trained on vast datasets that encompass a wide range of cultures and perspectives. However, it remains unclear which cultures and demographics are adequately represented and to what extent their perspectives are included in LLMs. In this topic block, we will analyze research papers that investigate and measure the inclusivity of various viewpoints within LLMs. We will discuss the methodologies used to assess cultural and demographic representation and the broader impact biased representation can have on different groups.
- Having Beer after Prayer? Measuring Cultural Bias in Large Language Models
- The Echoes of Multilinguality: Tracing Cultural Value Shifts during LM Fine-tuning
- Classist Tools: Social Class Correlates with Performance in NLP
- Sensitivity, Performance, Robustness: Deconstructing the Effect of Sociodemographic Prompting
- Subtle Biases Need Subtler Measures: Dual Metrics for Evaluating Representative and Affinity Bias in Large Language Models
- Large language models cannot replace human participants because they cannot portray identity groups
- Moral Decision Making with Language Models. Large Language Models (LLMs) have demonstrated the ability to pass various exams, ranging from US Law and Business School finals to the Written German State Examination in Medicine. This capability has led to proposals for using LLMs as assistants in important educational, legal and business decisions. However, if LLMs do not adhere to human morals, they may support unethical decisions and fail to understand human values. We will explore methods to assess the “moral compass” of LLMs and see if they are capable of performing moral reasoning.
- MoralBench: Moral Evaluation of LLMs
- Ethical Reasoning over Moral Alignment: A Case and Framework for In-Context Ethical Policies in LLMs
- Do Moral Judgment and Reasoning Capability of LLMs Change with Language? A Study using the Multilingual Defining Issues Test
- Rethinking Machine Ethics -- Can LLMs Perform Moral Reasoning through the Lens of Moral Theories?
- When to Make Exceptions: Exploring Language Models as Accounts of Human Moral Judgment
- Moral Foundations of Large Language Models
Through this seminar, students will gain a comprehensive understanding of the ethical and social dimensions of LLMs, preparing them to critically engage with these technologies in their future work.
- Representativeness of Language Models. Large Language Models (LLMs) are trained on vast datasets that encompass a wide range of cultures and perspectives. However, it remains unclear which cultures and demographics are adequately represented and to what extent their perspectives are included in LLMs. In this topic block, we will analyze research papers that investigate and measure the inclusivity of various viewpoints within LLMs. We will discuss the methodologies used to assess cultural and demographic representation and the broader impact biased representation can have on different groups.
Objectives
On the basis of suitable literature, in particular original scientific articles, students independently familiarize themselves with a topic in data-science, classify and narrow down the topic appropriately and develop a critical evaluation. Students work out concepts, procedures and results of a given topic clearly and with appropriate formalisms in a timely manner and to a defined extent in depth in writing; Evidence of independent development by presenting self-selected examples. Descriptive oral presentation of an in-depth data science topic using suitable media and examples in a given format.
Schedule
Registration
If you are interested in this seminar, please apply to Marlene Lutz via email.
Please start the Subject Line with “[SemDSII]”, and provide some details about your background, e.g., whether you have taken some relevant classes before and a short motivation to take this seminar. Also, make sure to indicate which of the two given topics you are interested in.