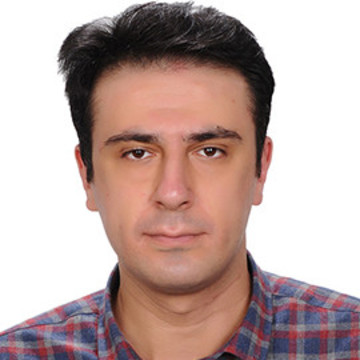
Prof. Siamak Khayyati, Ph.D.
Schloss Schneckenhof Ost SO 212
68161 Mannheim
My research interests are in design and control of production systems. In particular, I’m interested in developing methods for efficiently using partial information signals emitted from the production system in controlling the material release decisions.
Research Interests
Production systems consist of a multitude of machines and buffers connected in a network whose state information can be used for controlling the release of material into different parts of the system. Various control policies, e.g. the CONWIP and the Kanban policies, utilize predefined structures for using this information. Optimizing a control policy for every possible information signal can improve the performance of the system but it is often computationally prohibitive. As a middle ground solution, the set of information signals can be summarized into a smaller set with a manageable size. My research is partly focused on addressing the different aspects of this process, including identifying the signals, summarizing them and optimizing the policy parameters based on them, by using tools such as joint-simulation and optimization and machine learning.
Tan, B., Karabag, O., and S. Khayyati (2023): Production and energy mode control of a production-inventory system. European Journal of Operational Research 308, 1176-1187
Neghab, Davood Pirayesh, Siamak Khayyati, and Fikri Karaesmen. "An integrated data-driven method using deep learning for a newsvendor problem with unobservable features.“ European Journal of Operational Research (2022).
Khayyati, S. and B. Tan (2021): Supervised-learning-based approximation method for multi-server queueing networks under different service disciplines with correlated interarrival and service times. International Journal of Production Research.
Khayyati, S. and B. Tan (2021): A lab-scale manufacturing system environment to investigate data-driven production control approaches. Journal of Manufacturing Systems, 60, 283–297.
Khayyati, S. and B. Tan (2021): A machine learning approach for implementing marking-dependent production control policies. International Journal of Production Research.
Tan, B. and S., Khayyati (2021): Supervised learning based approximation method for single-server open queuing networks with correlated interarrival and service times. International Journal of Production Research.
Khayyati, S. and B. Tan (2020): Data-driven control of a production system by using marking-dependent threshold policy. International Journal of Production Economics.
Khayyati, S., A. Foroughi and R. Stolletz (2022): A Hybrid Approximation Method for Time-Dependent Queues in Service Systems, INFORMS APS 2023, Nancy, France, June 2023.
Khayyati, S. , Zenouzzadeh, S. M. and Stolletz, R. “A Machine Learning Approach to the Performance
Evaluation of Time-dependent Queues” XIIIth Conference on Stochastic Models of Manufacturing and
Service Operations, Autrans, France 2022
Tan, B. and Khayyati, S. “Performance Evaluation of Manufacturing Systems by Joint Use of Analytical Models, Simulation, and Supervised Learning” XIIIth Conference on Stochastic Models of Manufacturing and Service Operations, Autrans, France 2022
Khayyati, S. and B. Tan (2021): A machine learning approach for implementing data-driven production control policies. 40th Congress on Operations Research/
Khayyati, S. and B. Tan (2019): Data-driven control of a production system with partially observable correlated arrival processes. SMMSO 2019, Goslar, Germany, June 2019.
18th INFORMS Applied Probability Society Conference, Istanbul, Turkey, July 2015.
Education
2013 – 01/
2020
Doctoral Studies in Industrial Engineering & Operations Management (Barış Tan), Koç University, Istanbul, Turkey2013
Industrial Engineering (B. Sc.), Sharif University of Technology, Tehran, IranProfessional experience
10/
2021 – present
Postdoctoral Researcher, Chair of Production Management (Prof. Stolletz), University of Mannheim2020 – 08/
2021
Postdoctoral Researcher, College of Engineering, Koç University, Istanbul, Turkey2013 – 2020
Research Assistant, College of Engineering, Koç University, Istanbul, Turkey